Deep Diving into Deepfake
Explore the world of deepfakes with our in depth look at how advanced AI creates hyper realistic videos and the ethical implications involved.
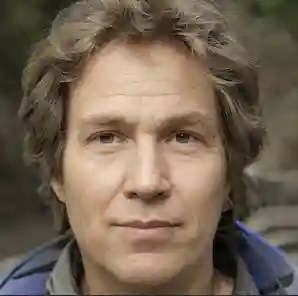
- Alexander Reed
- 6 min read
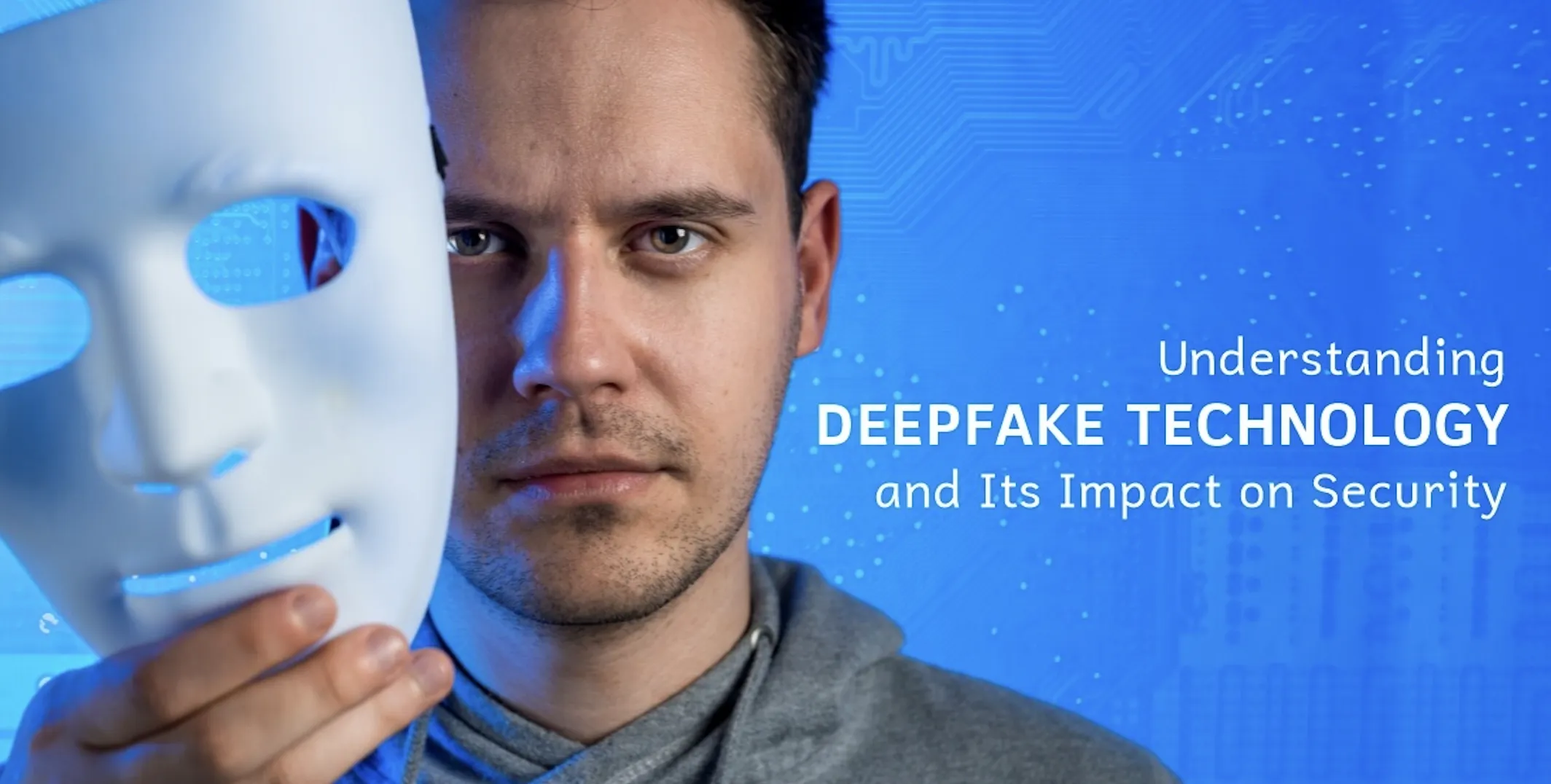
Hey there, tech enthusiasts! It’s your favorite blogger diving deep into the fascinating yet somewhat unnerving world of deepfakes. You’ve seen them, right? Those incredibly realistic and sometimes hilariously convincing videos where one person’s face is swapped onto another? Today, we’re not just scratching the surface; we’re plunging into the depths of how these deepfakes are crafted, the magic behind their realism, and the shadows that linger behind the technology.
Peeking Behind the Curtain: How Deepfakes Are Made
Ever wondered how your favorite comedian appeared to be giving a speech at the UN? Well, it all starts with something called deep learning, a type of artificial intelligence that’s as cool as it sounds. Let’s break it down:
Understanding Deepfake
video face swap free unlimited technology represents one of the most striking advancements in the field of artificial intelligence, leveraging complex machine learning models to create hyper-realistic video and audio content. This technology has the power to transform media, mimic human behaviors, and even challenge our perception of reality. At its core, deepfake utilizes sophisticated neural network architectures, specifically(GANs) and autoencoders. This article explores the intricate workings and principles of deepfake technology, breaking down the advanced techniques and algorithms that make it possible.
Neural Networks
At the heart of deepfake technology lie neural networks—systems of algorithms modeled on the human brain, designed to recognize patterns.interpret sensory data through a kind of machine perception, labeling, or clustering raw input. The architectures typically involved in deepfakes include Convolutional Neural Networks (CNNs) for detecting and manipulating faces, and more complex structures like autoencoders and GANs for generating new content.
Autoencoders
Autoencoders are a type of neural network used to learn efficient codings of unlabeled data. The network architecture comprises an encoder and a decoder. The encoder compresses the input and the decoder attempts to recreate the input from this compressed representation. In the context of deepfakes, autoencoders learn to capture the important features of a person’s face and reconstruct it with modifications as needed.
Generative Adversarial Networks (GANs)
GANs are the cornerstone of modern deepfake technology. They consist of two neural networks, the generator and the discriminator, which contest with each other in a game-theoretic scenario. The generator produces images that are as realistic as possible, and the discriminator evaluates them against a set of real images, learning to distinguish between the two. Over time, the generator learns to produce indistinguishable fakes, effectively “tricking” the discriminator.
The Deepfake Workflow
Data Collection and Preprocessing
The first step in creating a deepfake is to gather a substantial dataset of facial images or video footage of the target. This data is then preprocessed to align the faces, normalize lighting conditions, and crop them to a standard size. These preprocessing steps are crucial as they ensure that the neural networks train on data that is as uniform as possible, focusing on learning facial features rather than background noise.
Training Phase
Using the preprocessed data, the autoencoder or GAN begins the training process. For GANs, this involves the generator creating images that are passed to the discriminator along with a set of real images. The discriminator makes a judgment on each image’s authenticity, providing feedback to the generator. This process is iteratively repeated, with the generator continuously learning from the feedback to improve its fakes.
Optimization and Hyperparameters
The training process involves fine-tuning various hyperparameters such as the learning rate, the number of layers in the neural networks, and the activation functions. Optimizers like Adam or Stochastic Gradient Descent (SGD) are used to minimize the loss function, guiding the networks to better performance.
Crafting Your First Deepfake
If you’re thinking about trying your hand at creating a deepfake, here’s what you typically need:
- A hefty dataset of images or videos of the person you want to recreate. More data means more accuracy.
- Deepfake software: There are tools out there that can simplify this for beginners. For example:
- Patience and a powerful GPU: This process is a marathon, not a sprint, and requires some serious computational power.
The Art of Realism in Deepfakes
Creating a deepfake that can pass off as real is an art form. It’s not just about swapping faces; it’s about ensuring everything from the lighting and shadows to the subtlest movements of the face are perfect.
Matching Skin Tones and Lighting
One of the biggest giveaways of a deepfake is mismatched skin tones and inconsistent lighting. Advanced deepfake algorithms now incorporate techniques that adjust these aspects to ensure that the face not only fits the new head but matches its new environment too.
Facial Expressions and Movements
Ever seen a deepfake where the expressions were just… off? Getting the facial movements right is crucial. This is where sophisticated face tracking technology comes in, capturing everything from a frown to a smile and translating it onto the new face.
Exploring the Uses of Deepfakes: The Good, The Bad, and The Ugly
Deepfakes aren’t just for kicks and giggles; they have some seriously practical applications too. But with great power comes great responsibility, and not everyone’s intentions are pure.
Creative Arts and Beyond
In the movie industry, deepfakes can bring back yesteryear stars or improve the CGI of face replacements. Education sectors use them for interactive learning experiences, where historical figures come to life.
The Darker Side: Ethics and Misuse
Here’s the not-so-fun part. Deepfakes can be and have been used for creating false information, impersonating public figures, and even worse, non-consensual uses that can ruin lives. The ethics of deepfake technology are a battleground where innovation meets morality.
Navigating the Troubled Waters: Detection and Prevention
As deepfake technology evolves, so do the means to detect and regulate it. Here’s how the digital world is keeping up:
Detection Techniques: Seeing Through the Fakes
Advanced detection models are now trained to spot the subtlest signs of deepfakes. These include inconsistencies in blinking, facial hair, or even reflections in the eyes.
Legal and Social Safeguards
Laws are beginning to catch up, with countries implementing rules against malicious deepfakes. Social media platforms are also putting in safeguards to prevent the spread of potentially harmful deepfake content.
Wrap-Up: A Look Into the Future
The realm of deepfakes is expanding at an unprecedented pace. We’re heading towards a future where deepfakes could become everyday tools or potential threats. The development of deepfake technology poses important questions about reality, privacy, and trust in the digital age.
So, what’s your take? Are deepfakes a technological marvel, or do they spell trouble? Dive into the discussion below and let’s chat about the digital faces of tomorrow!